How is artificial intelligence shaping fintech?
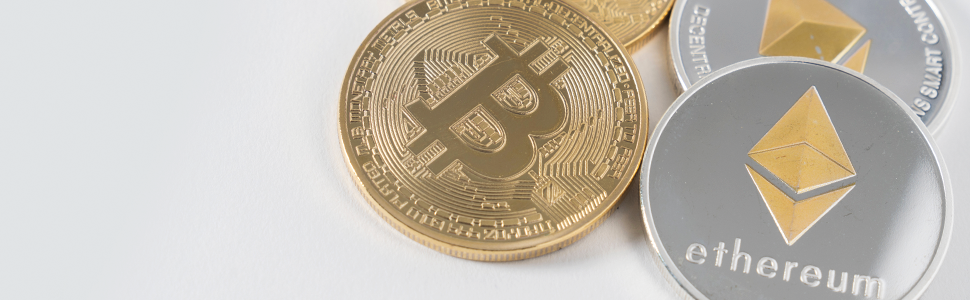
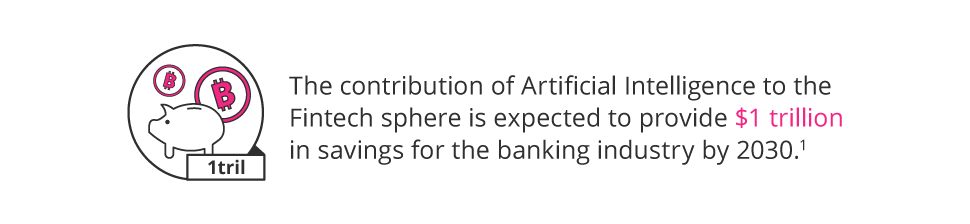
Furthermore, analysts are expecting financial automation to replace up to 47% of jobs across the industry.2 It’s clear AI will functionally impact all parts of the sector, with increasing importance and relevance to all those participating in the future of the financial industry.
There has been a rich history of actualising fintech AI: from the days of Talos defending Crete;3 to the chess-playing Mechanical Turk;4 to playing Go,5 and AI being the best performer on the stock market in 2017.6 With exponential improvements in the price and performance of computing power, storage, and internet bandwidth, AI is being applied to an increasingly broad spectrum of industries to solve a whole host of problems they face.7
AI brings remarkable value to organizations of all sizes across various industries. Learn how to take full advantage of these benefits with online artificial intelligence courses.
What is AI?
AI is any intelligence demonstrated by machines or computers, in contrast to the natural intelligence displayed by humans and other animals. Specifically in computer science, AI research is defined as the study of “intelligent agents”: any device that perceives its environment and takes actions to maximise its chance of successfully achieving its goals.8
In essence, AI consists of two main types: narrow AI and strong AI. Narrow AI is a computer program or learning algorithm designed to solve a very particular problem. For example, IBM Deep Blue beating multi-world champion chess champion Garry Kasparov; and more recently Alphabet (Google’s holding Company) becoming a Go Master in 21 days, winning all 100 of it’s qualifying games.9 In both cases, the AI was designed to compete at chess and Go respectively. If you asked either to perform a task outside of this – such as having a conversation about plants – they’d fail terribly.
On the other hand, strong AI refers to machines which are able to reliably pass the Turing Test (initially known as the Imitation Test) – which is to say they are indistinguishable from humans.10 For example, if you were given an instant messenger and told there was either a human or a computer on the other side talking to you, and you were unable to determine which it was – despite active questioning and engagement on a wide range of topics and activities (such as chess, logic arguments and general knowledge tests) – that AI is considered strong AI.
This was first (weakly) passed in 2014 by a chatbot called Eugene Goostman who achieved a 30% pass at the 60th anniversary of Turing’s death.11 There is significant debate around if or when it will become possible to achieve a reliable success rate.
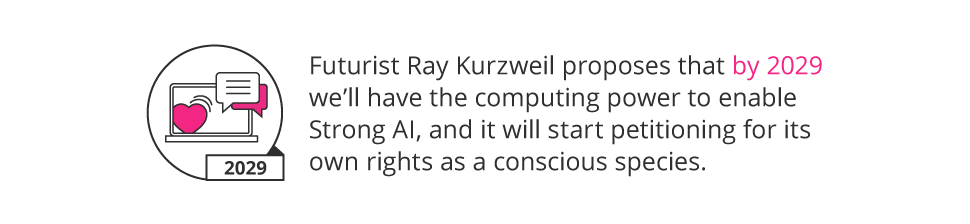
He further theorises that by 2040, AI will have in excess of all combined human cranial-computing power.12
The application of AI to the financial sector sees a clear departure from traditional models of financial innovation. Traditionally, the industry has evolved to market forces and iteratively improved their performance, customer experience, and net efficiency in the delivery of products and services. In contrast, disruptive fintech represents the leap forward that the financial tech industry is taking as a result of new technologies. These are often developed outside of the financial industry and are being applied to the sector. Among others, key technologies include: blockchain/distributed ledger technology, Big Data and AI (including machine learning), smart contracts, crowdfunding, Peer2Peer (P2P) lending and so forth.13
History of AI and finance
Going mainstream with high-frequency trading algorithms in 1998, and evolving into the disruptive fintech AI of today with highly customised and user-centric robo advisor services, such as Wealthfront and Betterment, it’s clear the financial sector is seeing rapid and disruptive advancement.14 The underlying technology itself is maturing, able to crunch enormous volumes of financial market data both quickly and intelligently to provide accurate measures of risk and high volumes of time-sensitive trades.
While the application of AI to the fintech development sector often focuses on the investment market, it’s important to note this has been a long process of evolution impacting many areas of the financial industry. To fully appreciate this, we need to cast our minds back to the 1980s.
This was the time when countries were mobilising to capture the rapidly growing computer market. Japan was busy investing $400 million in their “Japanese 5th generation Computer Project”,15 the UK’s Alvey Programme had raised £350 million,16 and the US’s Strategic Computing Initiative by Defense Advanced Research Projects Agency had raised over $1 billion,17 for the improvement of technology. This caused rapid growth, and it wasn’t long before the financial industry saw opportunities to leverage this new technology.
In 1986, the first real breakthrough was experienced in finance when K.C. Chen from the School of Business at California State University and Ting-peng Lian of the University of Illinois were able to correctly predict an 87-point drop in the Dow Jones Industrial Average through the use of an AI concept called, ‘’Expert Systems’’.18 Expert Systems, in essence, are computer programs or algorithms which aim to model specific human expertise in one or more narrow knowledge areas.
After a short, but tough AI winter between 1987 and 1993, improvements in the underlying technology – and with it, renewed funding – meant AI was again able to progress.19 By 1993, the industry was seeing opportunities to apply new fintech AI to the detection of fraud and the United States (U.S.) Department of Treasury’s funded FinCEN Artificial Intelligence system (FAIS) went live.20 In its first two years of operation, FIAS correctly identified over $1 billion in laundered funds.21
In 1998, the U.S. Securities and Exchange Commission authorised computerised high-frequency trading for the first time allowing for transactions that were thousands of times faster than humans to process. Just two years later, high-frequency trading accounted for 10% of all trades on the National Association of Securities Dealers Automated Quotations (NASDAQ).23
After a short, but tough AI winter between 1987 and 1993, improvements in the underlying technology -and with it, renewed funding – meant AI was again able to progress.
By 2010, trading times reduced from a few seconds per trade to mere nanoseconds, resulting in a dramatic increase in the percentage of AI-powered trades to 56% all NASDAQ trades.24 The application of fintech AI was in full swing, so much so that the world’s largest hedge fund – Ray Dalio’s Bridgewater Associates – managed to attract David Ferrucci – head of IBM Watson’s AI unit – away from the largest AI patent holder to participate in building market analysis, prediction, and trading financial tech tools based on AI.25
By 2016, investment had grown significantly with Google and Baidu spending an estimated $20-30 billion on their own internal research and development efforts, along with purchasing startups in the field of artificial intelligence.26 More specifically to finance, two of the top three performing hedge funds in 2017 were fintech AI-driven – the top performer drawing a 770% return over 12 months.27
Application of AI in fintech development
In present day, this rich history of disruptive fintech AI has sparked a series of investments in finance to explore the specific areas the technology can be applied in the industry. The fields of risk assessment, fraud detection and management, financial advisory services, trading, managing finance, and customer service have drawn particular interest.
Looking specifically at risk assessment, it’s worth first considering how this task is completed by a human in this function. Take, for example, a bank manager assessing a loan request: they request a host of information; review it; apply formal conditions and personal experience (gut sense); and then either approve or reject the loan, with an appropriate interest rate to offset the perceived risk. In the case of an AI, it’s able to complete exactly the same process, but with significantly more data from which to extract the demographics, psychographics, and other segment characteristics which determine loan repayment risk. Unlike the bank manager, the fintech AI learns from every loan the entire bank approves and rapidly learns from its mistakes as it attempts to optimise returns.
In present day, this rich history of disruptive fintech AI has sparked a series of investments in finance to explore the specific areas the technology can be applied in the industry.
From a fraud detection and management perspective, similar processes are associated with whether a bank should or shouldn’t process a payment, based on whether it passes advanced fraud prevention and anti-money laundering (AML) tests. In this case, all available historic data for the user, good actors (individuals who are not engaging in money laundering), and bad actors (individuals who have been found to be laundering in the past) are collected. By then using sophisticated learning algorithms to compare the specific situation against both the good and bad actor data, the institution is able to determine their confidence in the transaction being safe or not. In most cases, suspicious transactions are then escalated to an analyst to apply further human intelligence; however, they do so on 1% rather than 100% allowing for a far more thorough review.28
Robo advisors are an enormous business, growing 68% year on year and expecting to reach $2.2 trillion under management by 2020.29 Robo advisors are sophisticated AIs which look at historic stock performance, media, social media, and many other data sources with the aim of predicting future performance of stocks. The time horizon on this prediction ranges from 98 nanoseconds in the case of high-frequency trading, to many years in other forms of quantitative strategies.30 While some robo advisors focus on making actual trades based on the customer’s intended appetite for risk, others simply identify stocks for the user to consider for investment.
Back-office tasks are increasingly being automated by machines, to such a degree that research conducted by some banks have found 85% of their full-time staff could be replaced by machine automation in the coming years – a troubling point for a traditional employee.31 AI that engages in human conversation is not new. Originally developed in 1962 in the form of ELIZA then PARRY in 1972,32 their efficacy has improved significantly in recent years through refined algorithms, increased processing power and richer learning data.
When applied to customer relationship management or sales support, ChatBots are being taught to perform increasingly complex front-office tasks so that the vast majority of straightforward customer engagements are resolved in real time, 24/7. This would radically reduce the need for call centres, front-office staff, and other expensive customer-engagement resources.
What about unbanked and developing economies?
While fintech AI is clearly impacting the traditional first-world financial sector, it’s worth noting the novel approaches being taken to increase financial inclusion and facilitate efficient services to those in developing countries. Services such as Tala provide micro-finance to individuals based on their AI-crunched SMS history.33 In Kenya, DigiFarm34 has 19,000 farmers leveraging their agricultural produce to borrow as well as become better financially educated.35 In various other countries, AI is being leveraged to match P2P borrowers and lenders based on interests and risk profiles or appetite.
What of the future?
AI is expected to save the financial industry over $1 trillion in the next 17 years, an estimated current value of $1.2 trillion.36 Approximately 47% of financial jobs are at high risk of being replaced by automation.37 It’s clear AI is here to stay: with declining costs of computation, storage, and internet bandwidth combined with a projected 10-fold increase in volumes of data created daily.38
The prevailing question is how to navigate this incredible growth in new fintech AI-driven automation to ensure personal, business and industry sustainability? It’s clear the human component won’t be entirely removed, but the role will be redefined. Those who stay ahead of the curve will best leverage these new opportunities as the industry disrupts and reforms around Artificial Intelligence.
Invest in your future with fintech
- 1 Panzarino, H. (2018). “How is AI transforming financial institutions?” Retrieved from Fintech Futures
- 2 Rundle, M. (2014). “47% of all jobs will be automated by 2034, and ‘no government is prepared’ says Economist”. Retrieved from Huffpost
- 3Cummins, E. (2018). “Another AI winter could usher in a dark period for artificial intelligence”. Retrieved from Popular Science
- 4Morton, E. (2015). “The mechanical chess player that unsettled the world”. Retrieved from Slate
- 5 Gibbs, S. (2017). “AlphaZero AI beats champion chess program after teaching itself in four hours.” Retrieved from The Guardian
- 6 Lefton, H. (2017). “Top analysts are betting on these AI growth stocks”. Retrieved from CNBC
- 7 (2018). “Non-tech businesses are beginning to use artificial intelligence at scale”. Retrieved from The Economist
- 8 Poole, D., Mackworth, A., Goebel, R. (1998). “Computational intelligence: A logical approach”. Retrieved from Semantic Scholar
- 9 Gibbs, S. (2017). “AlphaZero AI beats champion chess program after teaching itself in four hours.” Retrieved from The Guardian
- 10University of Reading. (2014). Success marks milestone in computing history. Retrieved from Science Daily
- 11 Aamoth, D. (2014). “Interview with Eugene Goostman, the Fake Kid Who Passed the Turing Test”. Retrieved by Time Magazine
- 12 Reedy, C. (2017). “Kurzweil claims that the singularity will happen by 2045”. Retrieved from Futurism
- 13 (2018). “Oxford Fintech Programme”. Retrieved from GetSmarter
- 14 McCracken, H. (2016). “How Charles Schwab fought back against the robo-adviser startup invasion”. Retrieved from Fast Company
- 15Malik, R. (2002). “Japan’s fifth generation computer project”. Retrieved from ScienceDirect
- 16 (2018). “Appendix 4: Historic Government policy on artificial intelligence in the United Kingdom”. Retrieved from UK Parliament website
- 17 (2018). “Appendix 4: Historic Government policy on artificial intelligence in the United Kingdom”. Retrieved from UK Parliament website
- 18 Smith, C., McGuire, B., Huang, T., and Yang, G. (2006). “The History of Artificial Intelligence”. Retrieved from the Paul G. Allen School of Computer Science and Engineering
- 19 Cummins, E. (2018). “Another AI winter could usher in a dark period for artificial intelligence”. Retrieved from Popular Science
- 20 Senator, T., Goldberg, H., Wooton, J., Cottini, M., Khan, U., Klinger, C., Llamas, W., Marrone, M., and Wong, R. (1995). “The Financial Crimes Enforcement Network AI System (FAIS)”. Retrieved from Semantics Scholar
- 21 Senator, T., Goldberg, H., Wooton, J., Cottini, M., Khan, U., Klinger, C., Llamas, W., Marrone, M., and Wong, R. (1995). “The Financial Crimes Enforcement Network AI System (FAIS)”. Retrieved from Semantics Scholar
- 22 McGowan, M. (2010). “The rise of computerized high frequency trading: use and controversy”. Retrieved from Duke Law and Technology Review
- 23 McGowan, M. (2010). “The rise of computerized high frequency trading: use and controversy”. Retrieved from Duke Law and Technology Review
- 24 (2017). “History of High Frequency Trading (HFT) – Infographic”. Retrieved from Techstars
- 25 Joshi, A. (2016). “Rise of the machines: Artificial intelligence in the financial markets”. Retrieved from LinkedIn
- 26 Bughin, J., Hazan, E., Ramaswamy, S., Chui, M., Allas, T., Dahlström, P., Henke, J., and Trench, M. (2017). “Discussion Paper: Artificial intelligence the next digital frontier?” Retrieved from McKinsey Global Institute
- 27 Whyte, A. (2018). “Jersey-Based Bitcoin Fund Is 2017’s Second-Best Performer”. Retrieved from Institutional Investor
- 28 Varshney, K. (2017). “On the safety of machine learning: Cyber-physical systems, decision sciences, and data products”. Retrieved from Cornell University Library
- 29 Fraser, A. (2018). “Finance: Does AI mean the end for financial advisors?” Retrieved from Innovation Enterprise
- 30Zhang, Y. (2016). “Spectral analysis of high-frequency finance”. Retrieved from Massachusetts Institute of Technology
- 31 Dias, J., Patnaik, D., Scopa, E., and van Bommel, E. (2012). “Automating the bank’s back office”. Retrieved from McKinsey&Company
- 32 Merisalo, Sonja. (2018). “Developing a chatbot for Customer Service to Metropolia UAS Student Affairs Office”. Retrieved from Helsinki Metropolia University of Applied Sciences
- 33 (2018). “Giving credit where it’s due”. Retrieved from Tala
- 34 Strydom, C. (2017). “DigiFarm – Technology that bolsters change in Kenya”. Retrieved from Mezzanine
- 35 “Digifarm and connected farmer”. Retrieved from Safricom Twaweza
- 36Osborne, C. (2018). “Artificial intelligence will be worth $1.2 trillion to the enterprise in 2018”. Retrieved from ZDNet
- 37 Alam, N., and Kendall, G. (2017). ”Are robots taking over the world’s finance jobs?” Retrieved from The Conversation
- 38 Cave, A. (2017). “What will we do when the world’s data hits 163 Zettabytes in 2025?” Retrieved from Forbes