Detecting Cyberattacks Through the Ever-evolving Cybersecurity Industry
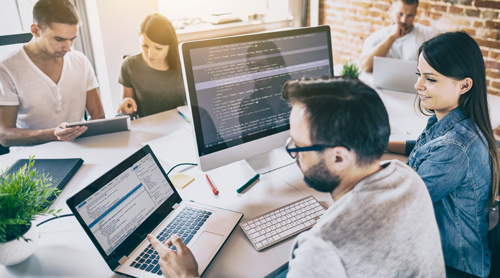
Cybersecurity has come a long way since its inception in the 1990s.1 In the beginning, hackers were generally considered a nuisance, and their hacks – which were pretty limited in terms of their scope – were designed more to cause trouble than harm.
When the internet began to take centre stage to businesses, and our lives – each new generation experiences increasingly sophisticated cyber attacks, and this brings about greater damage. The cybersecurity market and industry have had to evolve to effectively counter these attacks.
It all started here
It is generally accepted that the first created virus was called ‘The Creeper’, which infected ‘Arpanet’ – the foundation of the internet – in 1971.2 However, computer viruses and worms were predicted as early as 1949 by the mathematician John von Neumann, in his paper ‘Theory and Organisation of Complicated Automata,’ in which he proposed that a computer programme could reproduce.3
‘The Creeper’, was designed by Bob Thomas, an engineer who worked at BBN. The same company where email inventor, Ray Tomlinson worked. It wasn’t created to be malicious. In fact, infected machines would simply show the message, “I’m the creeper: catch me if you can,” but no damage was inflicted. In response to ‘The Creeper’s’ blatant challenge, the first antivirus software was created, know as ‘The Reaper’. It was also a self-replicating programme, which spread through the system in much the same way as ‘The Creeper’, and removed the offending virus from infected computers.
The term ‘computer virus’ was coined in the early 1980s when Fred Cohen, a PhD student at the University of Southern California, came up with the idea of using self-replicating software that spreads by attaching itself to existing programmes in order to attack the security of multi-user computing systems.4 When he showed his idea to Len Adleman, his thesis advisor, Adleman, remarked on how similar this principle is to a biological virus that uses the resources of the host cell it attacks to reproduce itself. The term ‘computer virus’ was born.5
The Morris worm creates huge impact
The Morris worm was released from an MIT computer by graduate student, Robert Tappan Morris at Cornell University in November 19886. It spread to internet-connected computers running the BSD variant of UNIX. Designed to be undetectable, a design flaw led it to create far more copies of itself than Morris estimated, resulting in the drastic over-taxing of all the computers it infected. This made it easy to detect, and made it possible to repair the back doors that it exploited.
When he showed his idea to Len Adleman, his thesis advisor, Adleman, remarked on how similar this principle is to a biological virus that uses the resources of the host cell it attacks to reproduce itself. The term ‘computer virus’ was born.
The Morris worm was not intended to be destructive, however, it caused computers to slow and buckle under the weight of unnecessary processing. The reason for Morris creating the worm is unclear; some speculate that the release was either premature or accidental.7 Regardless, the worm brought about two events that have become a major focus for those in IT.
First, Morris became the first person to be tried under the new Computer Fraud and Abuse Act of 1986, 18 U.S.C., section 1030(a)(5)(A).8 Morris was tried, convicted and sentenced to three years of probation,
400 hours of community service, a fine of $10,050, and the costs of his supervision.
Secondly, the Computer Emergency Response Team (CERT) was created by the Defense Advanced Research Projects Agency (DARPA) in the US. Their role was to coordinate information and responses to computer vulnerabilities, risks, and security.9
The evolution of viruses in applications, malware and bots
The early 2000s marked the rise of viruses being spread through applications (apps) – the third generation of malicious cyberattacks. Defensive strategies were evolving to combat cybersecurity attacks, but not as fast as the viruses were growing. What followed was the onset of malware, zero-day threats, bots, and other forms of attacks.
These gave rise to the following defensive strategies:
- Sandboxing
According to TechTerms this is a software management strategy that isolates applications from important system resources and other programmes and serves as another layer of security against malware or harmful applications penetrating systems.10
- Anti-bot
Bots interact with websites much the same way as humans, making them more difficult to detect. Bad bots perform activities such as web scraping, competitive data mining, personal and financial data harvesting, account takeover, digital ad fraud, spam and transaction fraud. They can cause significant harm to businesses. Anti-bot strategies differentiate between human and bot traffic, both bad and good, in order to isolate and terminate where necessary.11
Ransomware and beyond
The current fifth phase of cyberattacks is on a much larger scale, and even more invasive than the previous generations. Before ‘WannaCry’12 was detected in May 2017, we had never heard of the term ransomware. ‘WannaCry’, a trojan worm that exploited vulnerabilities in the Windows operating system – came with a two-pronged attack; delivering a self-propagating worm that locked up files in the host computer, as well as a ransom module that handled the ransom extortion activities. Bitcoin was its prefered method of payment, and once paid the files would be released.
One of these fifth generation attacks was carried out against USA’s retailer, Target, and saw the details of over 40 million credit cards hacked through the retailer’s point-of-sale systems.13 The attack was simply too advanced to have been identified or prevented by the industry giants based on the methods they were using at the time.
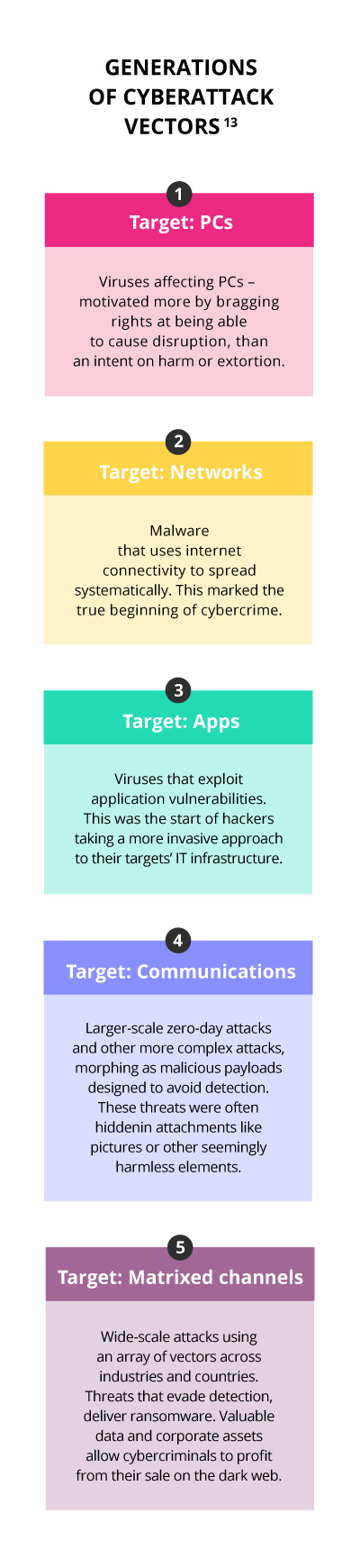
New technology for new challenges
The big antivirus companies established in the 1990s dominated the cybersecurity industry for nearly two decades, but with the rise of these large, well-organised attacks, new challenges arose, and with it came a demand for more innovative and creative technologies. The next generation of cybersecurity solutions are increasingly incorporating Artificial Intelligence (AI), machine learning technologies, and deep learning.15
The term AI first appeared in the 1950s16 to describe systems comprising a set of human-defined, “if or then” scenario decision rules. It is typically any system that performs tasks that have some semblance of automated decision-making.17 When used in cybersecurity, AI software analyses raw network activity data and flags unusual connections. This is basic pattern spotting and is fairly rudimentary.18 AI can also save analysts and companies from wasting time and money investigating false alerts by its ability to identify legitimate malicious activity.
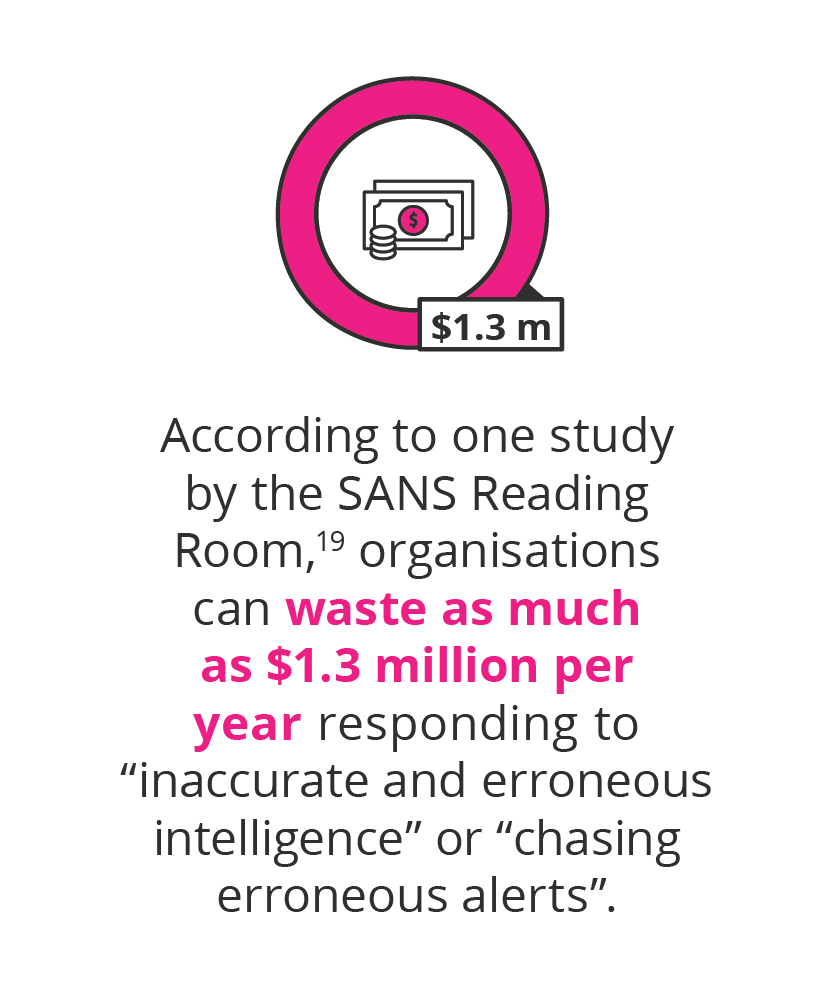
Machine learning (ML) is often expressed in the same breath as AI, but where AI is seen as a science, this is more of an approach.20 To learn from collected data, ML uses algorithms for prediction, classification, and insight generation through a formal body of methods grounded in solid mathematical foundations.21 Applied to cybersecurity, the right problems must be matched with the right ML tools.
- Deep learning
As a tool within machine learning, deep learning (DL) is highly dependent on matching the right problems to the right tools. Big technology companies like Google, Facebook, Microsoft, and Salesforce have already embedded DL into their products.
When it comes to cybersecurity, DL is proving its superiority over the rule-based and classic ML-based solutions when it comes to malware detection and network intrusion detection.22 Network intrusion detection systems are typically rule-based and signature-based controls that are deployed at the perimeter to detect known threats. Before DL, cyberattackers would get away with changing malware signatures and evading the traditional network intrusion detection systems.
DL is also effective when used to analyse a user’s behaviour over a series of actions in order to determine if something is out of the ordinary in their behaviour, and if a threat actor is using compromised legitimate network credentials, or if it’s an insider threat. For example, an employee accesses a local server that they hardly ever connect to, and suddenly start to download everything off the server. This approach is what is known as behavioural user analytics.23
In any of these cases, these technologies will recognise that there is an anomaly and will alert IT staff for further investigation. Behavioural user analytics is quickly becoming the gold standard for cybersecurity products in the AI domain.
The next generation of cybersecurity solutions are increasingly incorporating Artificial Intelligence (AI), machine learning technologies, and deep learning.
Another challenge the cybersecurity market is currently facing is the increased focus on data protection since the European Union’s enforcement of the General Data Protection Regulation (GDPR) in 2018.24 As a direct result of this regulation, many businesses are now compelled to implement further cybersecurity measures to keep the personal data of customers and employees safe. While this regulation only applies to companies that handle personal data from the EU, it is already transforming the cybersecurity stance of businesses across the globe.25
As cybersecurity threats continue to evolve and become more complex, the rapidly growing cybersecurity industry faces exciting new challenges as it looks to use cutting-edge cybersecurity detection tools, technology and techniques to fight the ever-present threat of malicious activity to keep user and business information safe.
- 1 (2018). ‘The fascinating evolution of cybersecurity’. Retrieved from LA Trobe.
- 2 Meltzer, T. (Oct, 2009). ‘From the first email to the first YouTube video: a definitive internet history’. Retrieved from The Guardian.
- 3 Rosenberger, R. (Nd). ‘When did the term ‘computer virus’ arise?’. Retrieved from Scientific American.
- 4 Rosenberger, R. (Nd). ‘When did the term ‘computer virus’ arise?’. Retrieved from Scientific American.
- 5 MacNeil, J. (Nov, 2018). ‘The computer virus is born, November 10, 1983’. Retrieved from EDN.
- 6 Kelty, C. (Nd). ‘The Morris Worm’. Retrieved from Limn.
- 7 Kelty, C. (Nd). ‘The Morris Worm’. Retrieved from Limn.
- 8 Curtis, T. (Nd). ‘Computer fraud and abuse act enforcement’. Retrieved from Digital Law.
- 9 (Nd). ‘What does CERT mean?’. Retrieved from TechoPedia.
- 10 (Jul, 2016). ‘Sandboxing definition’. Retrieved from TechTer.
- 11 (Nd). ‘What is an anti-bot?’. Retrieved from Distil Networks.
- 12 (May, 2017). ‘Ransom WannaCry’. Retrieved from Symantec.
- 13 McKoy, K. (May, 2017). ‘Target to pay $18.5M for 2013 data breach that affected 41 million consumers’. Retrieved from USA Today.
- 14 Rohlinger, L. (July, 2018). ‘The evolution of cybersecurity’. Retrieved from MSP Insights.
- 15 ‘Artificial intelligence for cybersecurity’. Retrieved from American Institute of Aeronautics and Astronautics.
- 16 Press, G. (Dec, 2016). ‘A very short history of AI’. Retrieved from Forbes.
- 17 Lin, D. (May, 2018). ‘Understanding the role of artificial intelligence, machine learning and deep learning in cybersecurity’. Retrieved from Exabeam.
- 18 (Nd). ‘Artificial intelligence for cybersecurity’. Retrieved from American Institute of Aeronautics and Astronautics.
- 19 Brown, R. (2019) ‘The evolution of cyber threat intelligence (CTI): 2019 SANS CTI survey . Retrieved from SANS Institute.
- 20 Polyakov, A. (May, 2018). ‘Machine learning for cybersecurity 101’. Retrieved from Towards Data Science.
- 21 Lin, D. (May, 2018). ‘Understanding the role of artificial intelligence, machine learning and deep learning in cybersecurity’. Retrieved from Exabeam.
- 22 Singh, S. (Jul, 2018). ‘Using the power of deep learning in cyber security’. Retrieved from Analytics Vidhya.
- 23 Wang, J. (Jun, 2017) ‘Deep learning in security’. Retrieved from DataBricks.
- 24 (Nd). ‘The EU General Data Protection Regulation (GDPR) is the most important change in data privacy regulation in 20 years’. Retrieved from EUGDPR.
- 25 Bagley, D. (Sep, 2018). ‘Insight into cybersecurity regulations is critical for today’s board members’. Retrieved from Law.